Bhargava Ramudu Kodela
The 9th edition of the Global Testing Retreat 2024!
About Speaker
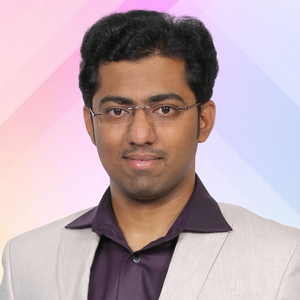
Bhargava Ramudu Kodela
Manager
_VOIS
Over 13 years of progressive experience as a Test Lead/ E2E Test Manager in Information Technology (IT) industry in Testing of Functional & Automation in RODOD & RSDOD (BSS & OSS) stack for telecom domain projects like System Integration testing – E2E involving entire Oracle components stack like Siebel CRM, Oracle BRM, Oracle AIA, OSM, ASAP & UIM.
● Technical skills on Billing & Rating functional Testing with OBRM system, Siebel CRM Testing and BSS system testing. Automation testing with JMeter & Selenium Web driver, OATS tool on automating Siebel Test cases & LISA DevTest Tool for Rest API & SOAP Web services automation.
● Transformation testing expertise in managing Portfolio Testing, System Integration Testing, API, E2E, UAT, Pre-Production testing, deployment verification, Cloud Migration testing, Defect Management for large scale transformation projects/programs.
More Speakers
Abstract in Detail:
These days Generative AI as tool is adopted & implemented for customer services and personalized customer workflows. It can respond more immaculate to customer for first contact resolution. So, with this in lieu we thought why not that we can have it in “Bug Analysis” i.e., debugging with Gen AI instead of manual debugging of logs.
Any E2E solution involves the integration between multiple components. So, while testing any E2E journey, whenever we encounter an error, as a primitive step we tend to check the “error description” based on which we can check the relevant application logs or the middleware integration layer logs connecting to the systems to figure out at which end the problem could be. This helps us to redirect the issue to the right application owner.
The idea here is to collect and maintain a repository of artifacts i.e., error codes, historic data of previously reported bugs with RCA and fix provided. This repository is then fed as an input to the GEN-AI. During the test execution phase (Automation/Manual) if any error is encountered, GEN-AI compares the error code with stored error codes and accordingly displays the root cause of the problem.
Hence with that output response, QA can directly approach the right application team to get this fixed instead of having multiple email threads to find the right application to fix the error.
Idea Outcome Result:
This introduction of GEN-AI will save at least 0.25 MD which is generally required to do the initial analysis, troubleshooting and identifying the right application.